Martin Leo, CISSP, shares his experience and insights in Singapore to help cybersecurity and risk professionals implement an AI governance framework, using an approach that will allow organizations to balance AI innovation with risk management.
The widespread adoption of AI by the financial services industry will offer significant transformative benefits, simultaneously exposing the institutions and the economy to new risks and, potentially, exacerbating existing risks.
Adoption of AI in the Financial Industry
Data publishing sites are widely used across the financial services industry, integrated into systems and processes – more so in AI systems today. Institutions will leverage AI to publish research and consume AI generated content – driving investing decisions. Yet in 2023, a site published AI-generated content that was found to contain multiple factual errors and inaccurate data about economic indicators. What happens when misinformation creeps into the systems and propagates through the financial networks? Will financial stability be at risk? How serious can it get?
The financial industry is experiencing an AI revolution with firms deploying AI everywhere from the front-office to the back-office. McKinsey estimates that AI technologies could potentially deliver up to $1 trillion of additional value each year. A BIS publication shows the main desired outcomes of AI use cases in financial institutions.
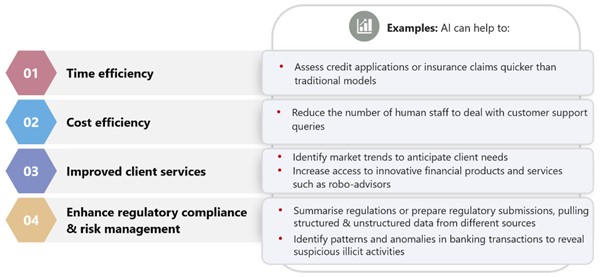
Risks of AI Use
Cybersecurity incidents can affect a financial entity and its ability to provide critical functions and services, ultimately affecting financial stability. Some 38% of respondents to a July 2024 European Banking Authority (EBA) Risk Assessment Questionnaire (RAQ) identified IT failures as the main driver of operational risk. Examples include:
- Errors in a bank's mortgage loan modification underwriting tool, which led to approximately 870 customers being incorrectly denied loan modifications or repayment plans. Many eventually lost their homes impacting their lives significantly.
- A coding error in an algorithm caused a trading firm to lose $440 million in 30 minutes.
Risks increase with the wider adoption of AI. Examples of potential risk:
- Credit risk: underestimating the probability of default due to inaccurate/incorrect data inputs
- Model risk: overestimating the capabilities of the model and using it in an inappropriate context
- Insurance risk: models not trained with recent disaster or crisis data, therefore unable to produce reliable pricing estimates
- Cyber risk: increase in vulnerability to cyberattacks due to increased attack surface area (increased interconnectivity with service providers)
- Other operational risk: system failure due to lack of interoperability with legacy systems
Authorities, including banking and finance industry regulators, have introduced AI-specific guidance around common themes such as reliability/soundness, accountability, transparency, fairness and ethics. In Singapore, the Monetary Authority of Singapore (MAS) published a report in December 2024, titled “Artificial Intelligence Model Risk Management – Observations from a thematic review”. The paper details good practices related to AI model risk management (MRM), observed during a thematic review of selected banks. The report contains a set of best practices that have been adopted by institutions and serves as a valuable reference for implementing robust AI governance.
Policy and Oversight
My view is that a policy for the governance of AI systems is the starting point to deliver a consistent message. Such a policy will require a review of existing policies to reflect the changing environment. Change management policies dating from the pre-AI era will require updating, to reflect a possibly democratized development community and an increasingly agile operational environment.
A cross-functional AI oversight forum to coordinate governance and oversight of AI use across the organization will also be beneficial in ensuring early risk identification and mitigation. Such a forum will also evaluate and advise on use cases that should not be pursued or pursued only after exhaustive review.
However, I also foresee that providing clarity on various terms and establishing the scope of controls will be a challenge for a policy author. Questions such as “What is an AI system” (vs “what is an AI model”) will need careful answering, to ensure controls are appropriately applied. Standards for securing a chatbot – should it address just the user interface, or the foundational models used or the underlying infrastructure in its entirety?
I find the EU AI Act and OECD.ai Policy Observatory a good reference for defining a system.
Inventory Management
To ensure AI systems are managed and monitored from the early stages and throughout their life cycle, AI systems/models should be used only after being registered in an inventory and approved for use. Existing inventory management tools can be used, providing a single, comprehensive repository.
Impact and Risk Assessment
A materiality assessment allows us to calibrate the approach to managing the risks. An AI system to manage office space (e.g., hot-desking) does not require controls at par with a mortgage loan decisioning system. Consider factors such as complexity, human in the loop or out of the loop. Inventory registration and impact assessment can be integrated into one flow.
Implement a Control Framework
The inherent complexities and risks of AI systems demand controls that extends beyond traditional software development practices, and which consider the entire lifecycle of an AI system. Organizations that proactively integrate risk management into AI development, validation and deployment will likely experience fewer issues down the line.
Data Management Controls
AI systems introduce unique challenges related to data integrity, fairness and performance, potentially generating biased or unreliable outputs. While existing data governance and management frameworks remain relevant, enhancing them for AI-specific use cases is crucial.
The data used for development and deployment must be assessed against standards established to ensure appropriateness, fairness and ethics. Enhance reliability by ensuring the training data is representative of the full range of conditions that the system will encounter in production.
Design your data processing workflows to incorporate rigorous quality checks to prevent bias, maintain integrity, preserve lineage and incorporates end-to-end security.
Proper documentation of data-related aspects, including data sources and lineage, further supports reproducibility and auditability, ensuring transparency in AI decision-making.
Model Selection
Aligning your chosen AI model with the intended objective is fundamental to achieving meaningful outcomes. Overly complex black-box models that lack transparency will make it difficult for you to achieve regulatory compliance and stakeholder trust. Testing for robustness and stability – through sensitivity analysis or stress testing – will help you mitigate some of these risks. Bias assessments, particularly for sensitive attributes, deserve your careful attention.
Validation
A structured validation framework will ensure that unintended consequences from your AI deployments are limited. The depth of validation should align with the system's materiality: higher-risk applications warrant more rigorous testing.
Segregation of duties continues to remain key: use a different team – separate from the development team – to undertake the validation. Third-party validation, especially for material impact system, will provide you with an extra layer of assurance. Security testing and adversarial testing play a crucial role, particularly as AI-driven threats become more sophisticated.
Deployment
Deployment controls will help to ensure your model performs as intended in real-world conditions. Pre-deployment validation in the production environment helps confirm that the system functions correctly and produces reliable results.
Monitoring
Performance metrics can be defined to monitor model degradation, model drift and other data issues, and to ensure that the model continues to perform as expected in a live environment. Appropriately designed fallback and contingency measures, such as kill switches and backup processes, will ensure disruptions can be effectively managed when AI systems malfunction.
Change Management
With AI models evolving rapidly, change management plays a pivotal role in maintaining system integrity. Implement proper version control, to avoid AI model updates becoming chaotic and leading to unintended consequences.
Third-Party Risk
Third-party AI introduces another layer of complexity for you to address. Many enterprise systems now embed AI capabilities from external vendors, raising concerns about transparency and governance. You can manage this risk better through a proactive approach, requiring disclosures from third parties and integrating AI performance monitoring into standard vendor management processes.
Cybersecurity considerations are also crucial, particularly when you are working with APIs, foundation models, or cloud-based AI services provided by third parties.
Takeaways for Risk Management Professionals
In advising organizations as they race to adopt and implement AI, it’s vital to balance AI innovation with robust risk management. As risk managers, we will need to consider and implement the following:
- Better monitor the challenges emerging as the use of generative AI and other AI models evolves
- Become aware of global standards and adjust policies accordingly
- Be extra-mindful of the risks that arise when AI is applied to cybersecurity solutions (e.g. SOC solutions, identity management)
- Learn from the past – end-user computing and model risk management challenges will continue as development is democratized
- Adapt cybersecurity threat models to evaluate threats due to AI systems (e.g., prompt engineering)
Martin Leo, CISSP, is Chief Risk Officer at the National University of Singapore. He has more than 20 years of experience in risk management including senior leadership roles in managing risk, including technology risk, at global banks. In his current role he considers the adoption of AI for risk management and is responsible for ensuring the necessary governance is in place for AI adoption.
Related Insights