Kapil Sharma, CISSP, CCSP
In an increasingly digitized world, the exponential growth of cyber threats has become an ever-pressing concern for individuals and organizations alike. My extensive experience in cybersecurity has equipped me with a solid understanding of the gravity of this situation. Over the years, I've witnessed firsthand how the relentless evolution of technology has given rise to increasingly sophisticated cyberattacks, rendering traditional security measures inadequate and outdated.
Drawing upon my cybersecurity experience, I recognize the pivotal role that Artificial Intelligence (AI) is playing in reshaping our response to these escalating threats. My hands-on involvement in implementing AI-driven solutions has allowed me to witness the transformational impact of machine learning, natural language processing, and data analytics. By integrating AI into cybersecurity practices, we can not only elevate the bar for threat detection and prevention but also proactively anticipate and mitigate emerging risks.
Using my own cybersecurity encounters as a basis, we will examine and clarify the symbiotic relationship between AI and cybersecurity. Along the way, we will gain a deeper understanding of how AI is reshaping the landscape and forging a new path in the ongoing battle against cyber threats.
By exploring various applications and benefits of AI in cybersecurity, we will also shed light on some of the dynamic strategies that are revolutionizing our defensive capabilities. From real-time anomaly detection to combating zero-day exploits, my involvement in this domain underscores the significance of AI in fortifying our digital infrastructure.
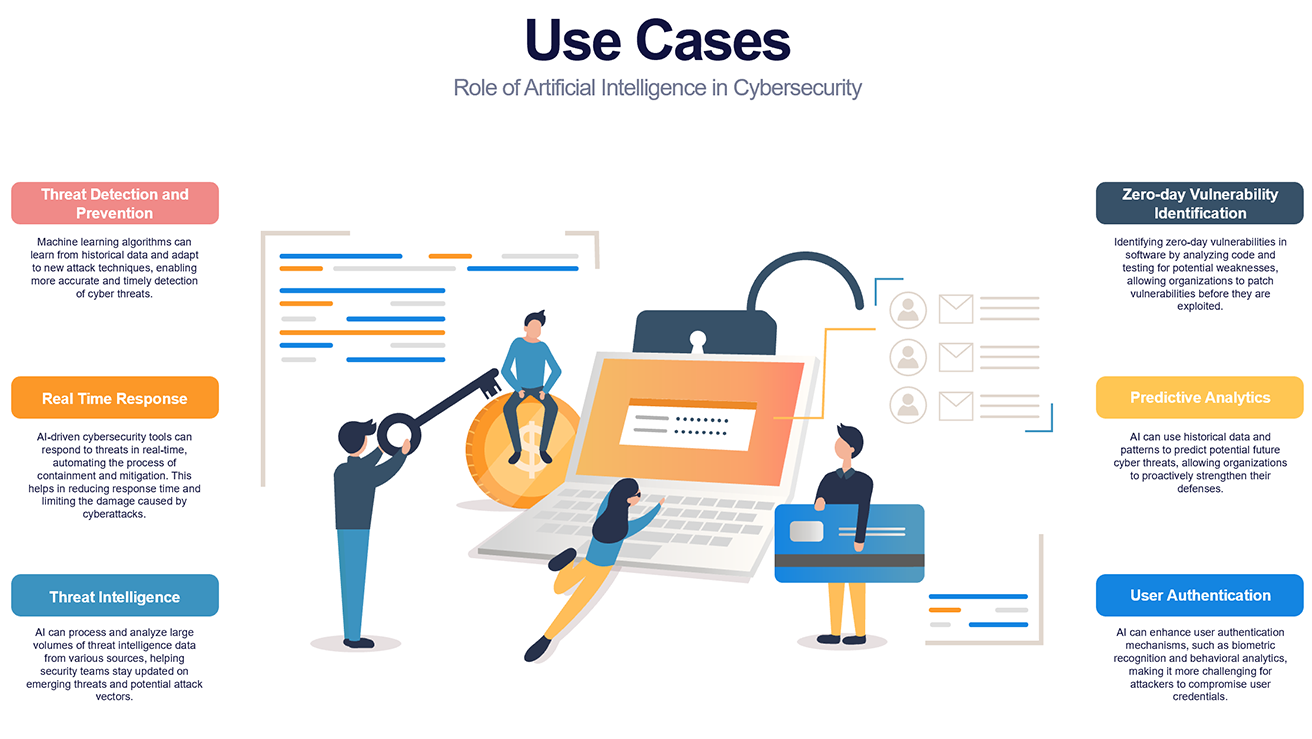
Threat Detection and Prevention
Using AI in threat detection and prevention requires a systematic approach that involves understanding the organization's cybersecurity needs, selecting appropriate AI technologies, integrating them into existing security infrastructure, and continuously improving the system.
Incorporating AI into threat detection and prevention strategies enables us to process massive volumes of data in near real time, discerning meaningful patterns and anomalies that elude traditional methods. Through AI-driven solutions, we can swiftly identify and respond to emerging threats, minimizing potential damages and safeguarding critical assets.
Real-Time Response
Having implemented AI-driven response systems, I've witnessed how these technologies facilitate the rapid identification of anomalies and deviations from established norms/ baselines. By continuously learning from data patterns, AI algorithms excel at discerning subtle irregularities that signify potential threats. This proactive approach allows organizations to thwart attacks in their nascent stages, preventing data breaches and minimizing operational disruptions.
Threat Intelligence
Leveraging AI in threat intelligence can enhance an organization's ability to effectively identify, analyze, and respond to emerging cyber threats. Implementation of NLP techniques to extract valuable information from unstructured data sources like news articles, blogs, forums, and social media can help in understanding human-generated threat intelligence content.
Zero-day Vulnerability Identification
AI can play a crucial role in identifying zero-day vulnerabilities by analyzing code and behavior. Extracting relevant features from the code and software binaries may require API calls, function signatures, control flow patterns and data dependencies. Integrating AI-based vulnerability identification system into the software development process can also benefit the detection of vulnerabilities by providing an additional layer of testing within the continuous integration/continuous deployment (CI/CD) pipeline.
Predictive Analysis
Predictive analysis using AI involves using historical data to make predictions about future events or trends. Once the AI model is trained and evaluated, use it to make predictions on new, unseen data. This is where the model applies its learned patterns to predict future events or outcomes.
Organizations need to think and evaluate carefully when selecting appropriate AI models for predictive analysis, such as regression models, decision trees, random forests, support vector machines, or deep learning models like neural networks.
User Authentication
AI can significantly enhance user authentication processes by analyzing various data points and behavior patterns to ensure secure and seamless access to systems and applications. There are ample AI technologies for user authentication, such as machine learning classifiers, anomaly detection algorithms, or deep learning models like recurrent neural networks (RNNs). Organizations needs to develop AI models that use behavioral biometrics, such as keystroke dynamics, mouse movements, or touchscreen interactions, to recognize individual users based on their unique patterns rather than their ability to provide a password or PIN when challenged.